SSS 03
List of all Book Series
Sigma Series in Stochastics
Previous Volume
|
|
Sigma Series in Stochastics -- Volume 3
Larger Picture
Jürg Kohlas / Paul-André Monney
Statistical Information.
Assumption-Based Statistical Inference
xii + 170 pages, soft cover, ISBN 978-3-88538-303-1, EUR 32.00, 2008
This book is a monograph that builds on the path-breaking work of Arthur
Dempster and Glenn Shafer, and before them R. A. Fisher, in the field of
statistical inference. The main thrust of the book lies around the idea
of statistical information. The inferential mechanism that is used in this
book to derive information about a parameter in a statistical experiment
is new, which is what sets it apart from other books on the topic. This
inference principle, which we call assumption-based reasoning, is based
on a sound combination of logic and classical probability theory. Some
traces of it can already be found in the original writings of Jacob Bernoulli,
but this book presents for the first time a much more complete and elaborate
descripton of it. In particular, it is shown that assumption-based inference
on functional models is a generalization of both Bayesian inference and
Fisher's fiducial inference. This is an interesting result regarding the
old controversy between these two theories. Our approach provides a new
and clear meaning to post-data probabilistic statements about an unknown
parameter, for example statements based on the likelihood function. In
particular, it is shown that this function cannot, in general, be considered
to carry the entire statistical information contained in the experiment.
Information about statistical experiments is described in this monograph by
functional models. They indicate how observations are generated in functions
of an unknown parameter and stochastic disturbances. In the first part of
the book we examine discrete functional models. These models are used to
present the basic ideas of assumption-based reasoning in a form that is
unhampered by technical difficulties. It is shown how several pieces of
information can be combined and how the result can be focused on a question
of interest. These operations provide an algebraic flavor to the analysis
of statistical information, which is a perspective that is presented here
for the first time. Some new preliminary results regarding a decision rule
for hypothesis selection are also presented. In the second part of the book
we treat several types of continuous models from the standpoint of assumption-based
reasoning. This allows us to review and clarify several concepts and difficulties
of Fisher's fiducial theory, for example the relation between traditional
confidence intervals and fiducial intervals. Our approach also permits to
determine the exact role and nature of improper priors in Bayesian inference.
Finally, the third part of the book is dedicated to the analysis of linear
models with Gaussian perturbations using assumption-based reasoning.
Contents
The following pdf-document contains the first and the second chapter in
full length to give you an impression of the contents of the work:
SampleChapters.pdf.
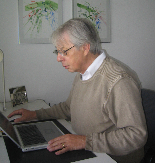 |
Jürg Kohlas is full professor of Computer Science at the University of Fribourg,
Switzerland. He directs the research group on Theoretical Computer Science. His
main research interests are inference under uncertainty, especially combining
logic and probability. Further, his interest cover logic and information,
including a theory of information algebras and uncertain information.
Homepage
Email: juerg.kohlas@unifr.ch
|
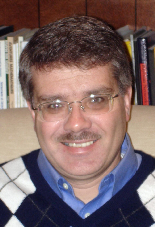 |
Paul-André Monney is a Consultant in Statistics and Information Fusion
based in Ely, Iowa, U.S.A. He was previously an Associate Professor of
Statistics at the University of Fribourg, Switzerland and a Visiting
Associate Professor of Statistics at Purdue University, Indiana, U.S.A.
He holds a Doctoral Degree in Mathematics and a Venia Legendi in
Statistics, both from the University of Fribourg, Switzerland. His main
research interests are computer science and statistics, in particular
Dempster-Shafer theory of evidence and theory of hints.
Homepage
Email: paulandre2000@msn.com
|

|